Definition: What is generative AI?
Generative AI refers to a category of AI systems that are designed to generate new data or content that is close to or even surpasses humans in their creativity. These systems use so-called neural networks to learn from existing data and then create new content. Essentially, these are algorithms that are able to be creative by recognizing patterns and using these patterns to create something new.
Is ChatGPT a generative AI?
Let's leave ChatGPT Answer that yourself: “Yes, I am a generative AI based on OpenAI's GPT-3.5 architecture. I was developed to generate human-like text based on the inputs provided to me and answer questions. ”
ChatGPT is probably the most well-known generative AI and is currently on everyone's lips. The ChatGPT developer “OpenAI” uses powerful language models such as GPT-3.5 and the even more powerful GPT-4, which can be used in a wide range of use cases, from answering questions and generating text to helping solve problems and communicate with users.
The difference between AI and generative AI
It is important to understand that there is no dividing line between artificial intelligence (AI) and generative AI. Rather, generative AI is a branch of AI that has made remarkable progress in recent years.
Most forms of AI are designed to classify or categorize existing data.
In contrast, the power of generative AI models is to generate completely new, even artistic, content that has never existed before. Generative AI therefore focuses specifically on creative tasks. This area has developed rapidly recently and plays a key role in today's AI landscape.
What is generative AI used for?
Generative AI has a wide range of applications, ranging from text generation to creative image production. There are now various providers and tools for this purpose that specialize in specific areas of application. We've listed a few examples in the table below:
These examples and descriptions illustrate how versatile generative AI can be used to find creative and efficient solutions. In addition to the providers mentioned above, there are now many different tools for every field of application from which users can choose.
How does generative AI work?
Generative AI models are based on advanced machine learning techniques such as transformers and diffusion models. These enable them to process extensive amounts of data. The focus is on two central architectures: diffusion and transformer models.
Diffusion models
Diffusion models, specialized in creating images, are a current key architecture. By training with images and associated descriptions, these models can generate new data patterns that are similar to the ones they were trained on.
The approach of diffusion models is to “deconstruct” training data by gradually adding so-called Gaussian noise and to transform it into a noisy image full of points. The model then attempts to recover the data by reversing this noise.
One is the generation of training data: In this case, artificial noise is added to images whose description is known. The aim of the training is for the model to reconstruct the original image as precisely as possible.
The actual application then consists of “pure noise” or an “image that does not match the description” to “re” construct an image that corresponds to the prompt, the description. You can think of it as a sculptor who starts with a marble block and removes everything from the marble block that doesn't look like the desired sculpture.
By the way, this technique works not only with images.
Transformer models
Transformer models are particularly used for tasks involving sequential data. This is data that has a specific meaning or relationships with each other, such as when processing natural language.
The transformer-based models use a special structure that uses “attention mechanisms.” These mechanisms assign greater importance to certain parts of the input data during processing. This allows them to work out and emphasize the meaning of a statement.
The GPT models use a special variant of the transformer, the so-called “transformer decoder”. It reads an entire sequence of data (for example a sentence) at once and can thus understand complex relationships between the words in a sentence. The models are trained on very large text data sets and then further refined specifically for specific tasks, such as translation, answering questions or text generation.
So much for the technical background. And what does that look like from the user's perspective?
Why does generative AI work so well in application?
Generative AI is pretty versatile in application: There are different types of input possible as a user — these can look different depending on the model. While so-called prompts are a common way to control the behavior of generative models, they can also respond to other types of input, such as images.
For many of the current models, a dialogue-based approach for controlling generative AI has prevailed. In this workflow, users or users interact with the model in a continuous dialogue, giving step-by-step instructions and responding to the generated output.
Let's take a closer look at how this process works:
- Formulation of the prompt: The user formulates a prompt in natural language. This prompt should be clear and precise so that the AI also delivers the desired result. For example, a prompt could read: “Write a short article about renewable energy and its impact on the environment.”
- Transfer of the prompt to the AI model: The formulated prompt is sent to the generative AI model. This can be done via an API or a special user interface provided by the provider. This is usually reminiscent of a chat interface.
- Processing through the AI model: The AI processes the prompt and uses its deep neural networks to learn patterns and information from the entered texts. She then tries to generate creative and relevant content based on these patterns and information. And that in a matter of seconds.
- Output generation: After the AI model processes the prompt, it generates a text response or other type of creative content that is presented to the user. The quality and relevance of the generated output depends heavily on the quality of the prompt.
- Feedback and iteration: In many cases, the user can now provide feedback and instruct the AI to refine or revise the output by formulating a new prompt. This process can be iterative to achieve the desired results.
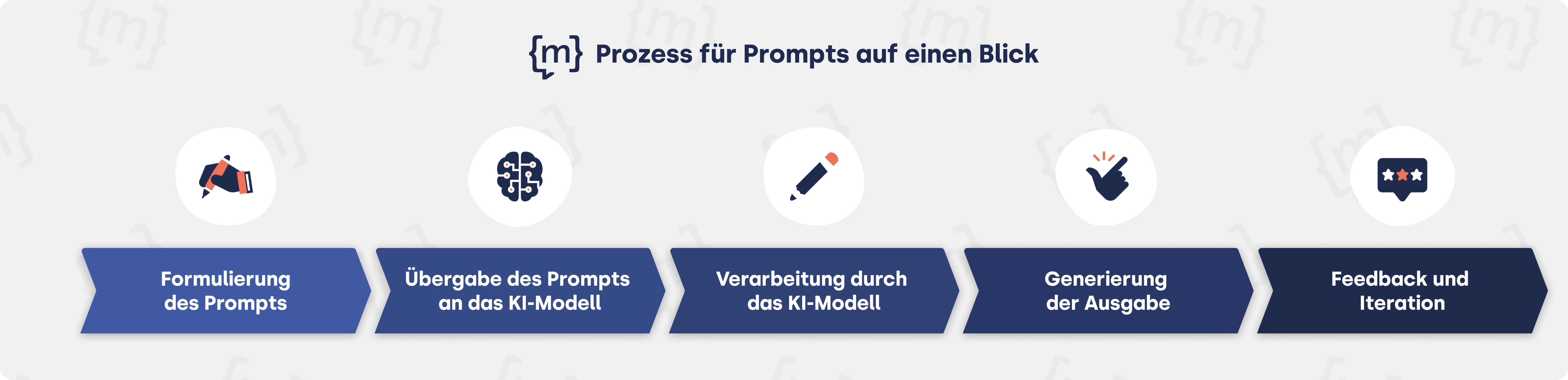
moinAIs Generative AI: Brilliant communication in the blink of an eye
We too at moinAI use generative AI in many places. We are currently using the technology in our new “Kompanion” feature. With this innovative tool, you can Chatbot answers create in seconds. You'll save time and rest assured that you'll get high-quality and creative answers. If the content creation previously took one to two weeks, it can be completed within a few hours with the company.
The company also goes beyond text generation and helps you create appealing response structures that positively influence the user experience. So that you can offer your users the ideal conversational design, the company always suggests three different conversational design concepts. For example, you can choose between a simple answer or an interactive answer with multiple choice options.
In addition, the company can analyze existing resources such as PDFs, websites or knowledge bases and generate suitable texts and the ideal structure from them. This allows you to make optimal use of existing content.
The answers generated are always customizable so you can be sure that they meet your needs and standards.
In short: With moinAI's Kompanion, you have a powerful tool at your disposal to take your communication to the next level.
Conclusion & outlook
Generative AI has revolutionized the world of creativity and automation. From text generation to image and music composition to medical diagnosis and image processing, this technology opens up exciting new possibilities.
With ever more powerful generative AI models, the fields of application will continue to grow and expand our capabilities for creative and productive work. The future of generative AI promises exciting developments and innovations in almost all industries. As you can see, it is time to say goodbye to the idea that AI is only suitable for analytical tasks.
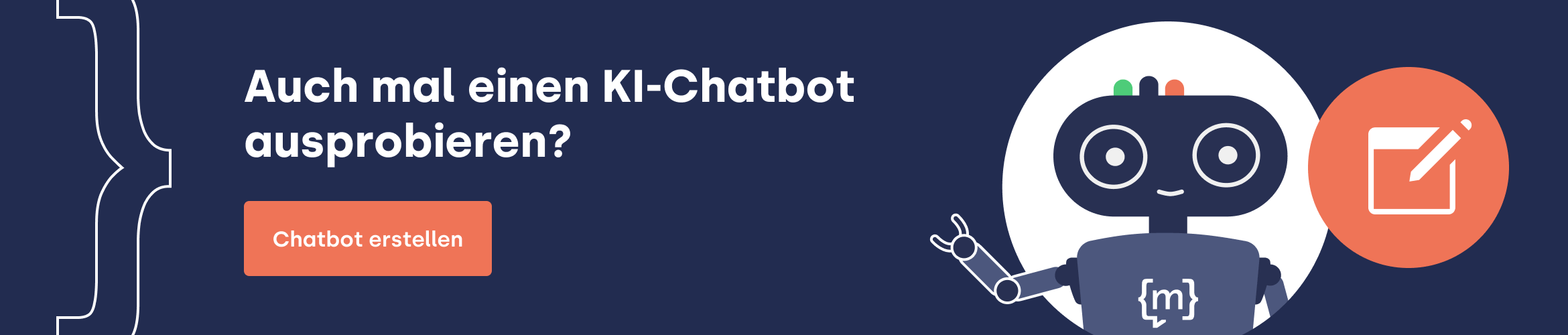